Sales Forecast Methods β How to Predict Your Sales for Permanent Growth
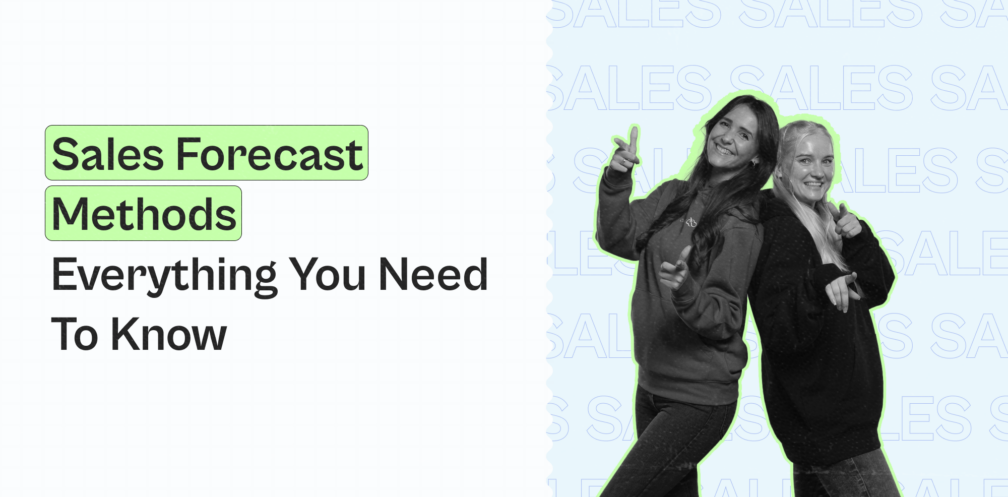
Sales forecasting is super important for businesses because it helps them plan and make smart decisions. They want to know how much they’ll sell in the future, so they use different methods to figure it out. It combines looking at numbers and using people’s opinions and knowledge.
In this article, we will dive into the world of sales forecast methods and explore the important numbers that can give you valuable insights about your sales. When you understand and use these numbers, you can get ahead of the competition, improve your sales strategies, and grow your business.
So come join us to learn more about sales forecasting methods, metrics and tools. Get ready to boost your sales game!
The importance of sales forecasting for outbound sales
Sales forecasting plays a crucial role in driving success and growth for businesses, particularly in outbound sales. By accurately predicting future sales trends and customer demand, companies can make informed decisions and optimize their strategies.Β
Here are the key reasons why sales forecasting holds immense significance for outbound sales:
- Resource allocation: Effective sales forecasting enables organizations to allocate their resources efficiently. By understanding anticipated sales volumes, companies can allocate their budgets, staff, and inventory to meet future demands, ensuring optimal utilization of resources.
- Budgeting and financial planning: Sales forecasting provides vital insights for budgeting and financial planning. It helps businesses determine revenue projections, set achievable targets, and make informed investment decisions. By aligning sales forecasts with financial planning, companies can effectively manage cash flow, control costs, and make strategic investments.
- Sales pipeline management: Accurate sales forecasting allows for better management of the sales pipeline. It helps identify potential bottlenecks, streamline processes, and optimize lead generation and conversion rates. Sales teams can prioritize their efforts based on the forecasted pipeline, enhancing productivity and maximizing sales opportunities.
- Performance evaluation and accountability: Sales forecasting serves as a benchmark for performance evaluation and accountability. By comparing actual sales figures with forecasts, organizations can assess individual and team performance, identify areas for improvement, and establish realistic sales targets. It fosters accountability among sales professionals, driving motivation and ensuring continuous growth.
By leveraging accurate forecasts, businesses can enhance their strategic decision-making, optimize sales processes, and achieve long-term success in a competitive marketplace.
Common sales forecasting methods
Two primary approaches used in sales forecasting are qualitative and quantitative methods. Understanding the characteristics and considerations of both sales forecasting models allows you to make more informed decisions to support your strategic planning and operational activities.
Let’s explore qualitative and quantitative methods in more detail, examining their key features, applications, strengths, and limitations.
Qualitative sales forecasting methods
Qualitative sales forecast methods rely on subjective opinions and expert judgment rather than statistical analysis or quantitative data.
Sales and marketing teams commonly use the following sales forecast methods to make more informed decisions on the next steps to take:
- Opportunity stage forecasting
- Multivariable analysis forecasting
- Length of sales cycle forecasting
- Intuitive forecasting
Let’s look at these techniques, focusing on their benefits and downsides.
Advantages and disadvantages of using qualitative sales forecast methods
Opportunity stage forecasting
Opportunity stage forecasting is a special way of predicting how likely it is to make a sale at different points in the selling process. Instead of guessing how much they will sell overall, businesses can look at each deal individually to see how likely it is to happen.
Using opportunity stage forecasting helps businesses understand their sales process better. It gives them essential information about how likely they are to make a sale at different steps along the way. This helps them make smart choices to improve their sales.
For example:
Imagine a company that develops and sells project management software to other businesses. Their sales process includes multiple stages:Β
- prospecting,Β
- product demo,
- negotiation,Β
- and contract signing.
With opportunity stage forecasting, the company can assess the probability of closing deals at each stage. For instance, let’s say they have 10 potential customers in the negotiation stage. By analyzing historical data, they find that, on average, 7 out of 10 prospects in this stage end up signing contracts.
Using this insight, the sales team can make informed decisions.Β
They may allocate more resources to high-probability opportunities, such as assigning additional salespeople or providing extra support. At the same time, they can identify deals with a lower chance of success and take appropriate actions, such as offering incentives or adjusting the sales strategy, to improve the probability of closing those deals.
By focusing on the most promising opportunities, the company can optimize its sales efforts, allocate resources efficiently, and increase its chances of generating revenue.Β
Now letβs review the main pros and cons of this method:
Pros:
- Its benefits include prioritizing sales teamsβ efforts since it focuses on opportunities that are more likely to close.
- Since it considers the different stages of the sales process, its forecast is more accurate.
- It helps sales managers identify bottlenecks in the sales process and take action to correct them.
Cons:
- It requires a well-defined sales process with clearly defined stages, which is not feasible for many businesses.
- Tracking and updating opportunities at each stage of the sales process is also time-consuming.
- It doesnβt consider external factors like the competitive landscape or changes in the market, which can impact the sales process.
Multivariable analysis forecasting
Multivariable analysis is a sales forecasting model that uses multiple variables or factors to predict future sales performance. Instead of relying on a single input variable, such as historical sales data, this method considers numerous variables that may influence sales, such as market trends, customer behavior, economic indicators, and marketing campaigns.
Multivariable sales forecasting can be more accurate than other methods because it considers a broader range of factors that may affect sales. However, developing and interpreting the forecasting models may also require more sophisticated statistical techniques and expertise.
For example:
Let’s consider a B2B SaaS company that provides businesses with customer relationship management (CRM) software. They want to forecast their future sales performance using multivariable analysis. Here’s an example of how they can apply this method:
- The company starts by gathering various data points related to its sales performance and market conditions.Β
- They consider factors such as historical sales data, market trends in the CRM software industry, customer behavior and preferences, economic indicators (e.g., GDP growth, industry-specific economic indicators), and the impact of their marketing campaigns.
- Using multivariable analysis, the company develops a forecasting model incorporating all these variables.Β
- They use sophisticated statistical techniques to analyze the relationships and correlations among the different factors.Β
For example, they might find that increasing market trends and positive customer sentiment lead to higher sales.
Once the model is established, the company can use it to predict future sales performance. They input the current values of the various variables into the model, such as the current market trends and customer behavior.Β
The model then forecasts their future sales, considering the combined influence of all the factors.
Now letβs consider the pros and cons of this sales forecasting method:
Pros:
- It provides a more comprehensive and nuanced understanding of the factors influencing sales. By considering multiple variables, businesses can capture the complex interplay of various factors and make more accurate predictions.
- It also enables businesses to identify the relative importance of different variables in driving sales, allowing for targeted decision-making and resource allocation.
Cons:
- Requires careful selection and measurement of independent variables and robust data collection and analysis, so it is more challenging to implement than other sales forecasting techniques.
- Collecting and analyzing data from multiple sources can be time-consuming.
Length of sales cycle forecasting method
The Length of Sales Cycle forecasting method estimates the duration or length of time it takes for a sales opportunity or deal to progress from the initial contact with a prospect to the final closing of the sale. It focuses on understanding and predicting the timeline or cycle of the sales process.
For example:
Think of a B2B SaaS company offering businesses project management software. They want to forecast the length of their sales cycle, which is the time it takes from the first contact with a prospect to closing the sale.
- The company starts by analyzing historical data of its past sales cycles.Β
- They gather information on the average time it took for deals to progress through each stage of the sales process, such as initial contact, product demonstration, negotiation, and contract signing.
- Using this data, they develop a forecasting model that estimates the expected duration for each sales cycle stage. For instance, they might find that on average, it takes three weeks from the initial contact to the product demonstration, two weeks from the demo to negotiation, and another two weeks from negotiation to contract signing.
- Based on this historical analysis, the company can forecast the length of future sales cycles. For instance, if they have a new prospect in the initial contact stage, they can estimate the expected timeline for that deal to progress through the remaining stages and close the sale.
By accurately forecasting the length of sales cycles, the company can better plan its resources and manage customer expectations.Β
Letβs review the main pros and cons of this method:
Pros:
- It allows companies to determine the number of sales reps needed, plan marketing campaigns, and manage cash flow more efficiently.
- It assists in managing the sales pipeline by identifying potential bottlenecks or areas where leads tend to get stuck. It enables businesses to address these issues and optimize the sales process.
Cons:
- If data is limited or unreliable, the forecast may not accurately reflect the future sales cycles.
- This method may not account for external factors that can impact the sales cycle, such as changes in market conditions, competition, or customer behavior.
Intuitive forecasting method
Intuitive forecasting, or judgmental forecasting, is a qualitative method that relies on the expertise, experience, and subjective judgment of individuals or a group of experts to predict future sales or business outcomes. It involves using intuition, personal knowledge, and insights to make informed forecasts rather than relying solely on quantitative data or statistical models.
For example:
Imagine a B2B SaaS company that provides customer support software to businesses. They want to forecast their future sales or business outcomes using Intuitive Forecasting.
- The company gathers experts, such as sales managers, customer success managers, and product specialists.Β
- These individuals deeply understand the market, industry trends, customer behavior, and the companyβs product offerings.
- During an Intuitive Forecasting session, the experts share their insights and discuss the factors that may impact future sales or business outcomes.Β
- They use their collective knowledge, experience, and intuition to make informed forecasts.
For example, they might consider the current market conditions, such as the level of competition, emerging industry trends, and the economic landscape. They might also discuss customer feedback and preferences, potential changes in customer needs, and the impact of new product features or enhancements.
Based on these discussions and the participants’ expertise, the company generates intuitive forecasts for future sales or business outcomes.Β
These forecasts are subjective in nature but are grounded in the deep understanding and insights of the experts involved.
The intuitive forecasts can then inform strategic decision-making within the company.
Letβs consider its benefits and downsides:
Pros:
- It leverages the knowledge and expertise of individuals with domain-specific education or experience. It can provide valuable qualitative insights impossible to capture by quantitative data alone.
- Intuitive forecasting allows for flexibility and adaptability to changing market conditions, as experts can incorporate real-time information and adjust their forecasts based on their understanding of the business environment.
Cons:
- Since intuitive forecasting relies on individual judgment, forecasts may vary significantly among different experts or stakeholders.
- Intuitive forecasting is subjective in nature and can be influenced by personal biases, assumptions, or individual perspectives.
Quantitative sales forecasting methods
Quantitative sales forecasting methods are mathematical models that use historical data to generate sales forecasts.
These methods are helpful when historical data is available and can provide valuable insights into past sales trends and future sales outcomes.Β
However, they may not be as effective when past data is limited or it is difficult to quantify factors that influence sales, such as changes in consumer behavior or unexpected events.
Some common quantitative sales forecasting methods include:
- Time series analysis
- Regression analysis
- Exponential smoothing
Letβs look closer at these methods:
Time series analysis
This method uses historical data to identify patterns and trends over time. You can use it to forecast sales based on past sales data and factors that may impact sales, such as seasonality or economic trends.
Time series analysis can be particularly useful in situations when:
- A sufficient amount of data is available
- Sales patterns are consistent over time
- Limited external factors are impacting sales
- For short-term forecasting
For example:
Imagine a B2B SaaS company that offers HR management software to businesses. Using the Time Series Analysis method, they want to forecast their sales for the upcoming quarter. They have access to historical sales data for the past several years and want to identify patterns and trends to predict future sales.
- The company gathers its sales data, which includes information about the number of new customers acquired and the revenue generated each month over the past few years.Β
- They also consider external factors such as economic indicators, industry trends, and significant marketing or promotional activities during that period.
- Using Time Series Analysis, the company analyzes historical sales data to identify patterns and trends.Β
- They look for recurring patterns, such as regular fluctuations or seasonal trends, and assess how these patterns may impact future sales. For example, they may notice that sales tend to increase during certain months due to the nature of their target market or the demand for their software.
- Once the patterns and trends are identified, the company can use the historical data to project future sales.Β
- They take into account the historical patterns and any anticipated changes in external factors that may influence sales, such as economic conditions or upcoming industry events.
Based on the Time Series Analysis, the company can make short-term sales forecasts for the upcoming quarter.Β
Now letβs consider the main pros and cons of this method:
Pros:
- Reveals patterns and trends: Time series analysis helps identify patterns and trends in historical sales data, allowing businesses to understand past performance and make informed predictions about future sales.
- Incorporates factors affecting sales: By considering factors such as seasonality, economic trends, and other external influences, time series analysis provides a more comprehensive view of sales forecasting, resulting in more accurate predictions.
- Useful for short-term forecasting: Time series analysis is particularly effective for short-term forecasting, providing businesses with insights into immediate sales trends and allowing them to make timely adjustments to their strategies.
- Provides a baseline for comparison: By analyzing historical data, businesses can establish a baseline for sales performance and compare current sales figures against expected values, enabling them to assess their progress and identify areas for improvement.
Cons:
- Limited applicability in volatile markets: Time series analysis assumes that sales patterns remain consistent. However, historical data may not accurately reflect future sales trends in highly volatile markets or industries with rapidly changing conditions.
- Incomplete analysis without considering external factors: While time series analysis can capture seasonal patterns, it may not account for all external factors that impact sales, such as changes in competition, market trends, or technological advancements. Incorporating these factors requires additional analysis or other forecasting methods.
- Reliance on historical data accuracy: The accuracy of time series analysis heavily depends on the quality and reliability of historical data. Inaccurate or incomplete data can lead to inaccurate forecasts and unreliable insights.
- Difficulty in capturing unexpected events: Time series analysis is less effective in capturing sudden or unforeseen events that can significantly impact sales. It may struggle to account for unpredictable factors such as natural disasters, economic crises, or disruptive industry developments.
Regression analysis forecasting
Regression analysis identifies relationships between two or more variables and generates forecasts based on those relationships.
Regression analysis can be helpful in situations when:
- Multiple factors impact sales
- Historical data is available
- Sales patterns are not consistent over time
- For long term-forecasting
- To identify long-term relationships between variables.
For example:
A B2B SaaS company offers a project management tool and wants to forecast its sales for the next five years using Regression Analysis. They have access to historical sales data and data on various factors that may impact sales, such as marketing expenses, customer satisfaction scores, and website traffic.
- The company gathers historical sales data for the past five years and collects information on the aforementioned factors for the same period.Β
- Using Regression Analysis, they can analyze the relationship between these variables and sales to uncover any significant correlations. For instance, the analysis may reveal a positive relationship between marketing expenses and sales. As marketing expenses increase, sales tend to increase as well. Similarly, they may find that higher customer satisfaction scores and increased website traffic are associated with higher sales.
- The company can develop a regression model that predicts sales based on the identified factors by understanding these relationships.Β
- They can then use this model to forecast sales for the next five years by inputting the expected values of the factors.
Regression Analysis is particularly useful in situations where multiple factors impact sales.Β
Letβs take a look at the main pros and cons of this method:
Pros:
- Identifies relationships between variables: Regression analysis helps identify and quantify relationships between multiple variables and sales. It provides insights into how changes in factors such as marketing expenses, customer satisfaction, or website traffic impact sales, enabling businesses to make data-driven decisions.
- Quantifies the impact of variables: By utilizing regression analysis, businesses can quantify the impact of each variable on sales. This allows for a better understanding of which factors have the most significant influence on sales performance, helping prioritize resources and focus efforts on the most impactful areas.
- Provides long-term forecasting: Regression analysis is well-suited for long-term forecasting, as it identifies and models relationships between variables over an extended period. This helps businesses plan for the future, set realistic goals, and make strategic decisions to achieve sustainable growth.
- Utilizes historical data: Regression analysis relies on historical data, making it valuable when sufficient data is available. By leveraging past sales data and associated factors, businesses can create robust forecasting models considering trends and patterns observed over time.
Cons:
- Assumes relationships are linear: Regression analysis assumes a linear relationship between the independent and dependent variables (sales). However, in reality, relationships can be more complex, and nonlinear patterns may exist. Failing to capture nonlinear relationships may result in inaccurate forecasts.
- Requires accurate and complete data: Regression analysis heavily relies on the quality and completeness of historical data. Inaccurate or incomplete data can lead to biased forecasts and unreliable insights. Ensuring data accuracy and integrity is crucial for obtaining meaningful results.
- Limited by available variables: The accuracy of regression analysis depends on the availability of relevant variables that impact sales. If important variables are not included in the analysis, it may overlook significant factors that influence sales performance, leading to less accurate forecasts.
- Vulnerable to outliers and anomalies: Regression analysis can be sensitive to outliers or anomalies in the data. Unusual or extreme data points can skew the results and affect the accuracy of the forecasts. Careful data preprocessing and outlier detection techniques should be employed to mitigate this risk.
Exponential smoothing
Exponential smoothing is a historical forecasting method that uses a weighted average of past sales data to generate a forecast. It is useful for:
- short-term forecasting
- smoothing out short-term fluctuations
- forecasting sales when sales patterns are consistent over time
For example:
Imagine a B2B SaaS company that offers a subscription-based LinkedIn automation tool (just like us ). They want to forecast their monthly sales for the next quarter using the Exponential Smoothing method. They have access to historical sales data for the past two years.
Using Exponential Smoothing, the company can analyze the historical sales data and calculate a weighted average to generate a forecast.Β
The method assigns higher weights to more recent sales data, capturing the most relevant trends and patterns.
For instance, let’s assume that the company’s sales have been relatively consistent over time, with some short-term fluctuations due to seasonality or promotional campaigns. Exponential Smoothing can be employed to smooth out these short-term variations and generate a reliable forecast for the upcoming months.
By applying the Exponential Smoothing method, the company can accurately predict their sales for the next quarter based on the weighted average of past sales data.Β
The forecast will consider recent trends and patterns, giving the company a better understanding of expected sales performance.
Exponential Smoothing is particularly useful for short-term forecasting, where sales patterns are consistent over time.Β
Now letβs talk about its benefits and downsides:
Pros:
- Effective for short-term forecasting: Exponential smoothing considers recent sales data more heavily, allowing businesses to anticipate sales levels in the upcoming period.
- Smoothing out short-term fluctuations: This method filters out temporary disturbances, such as seasonal fluctuations or irregular promotions, allowing businesses to focus on the underlying patterns.
- Simple and easy to implement: Exponential smoothing requires minimal computational complexity and can be applied using spreadsheet software or specialized forecasting tools.
- Efficient utilization of historical data: By leveraging past data, businesses can use available information to predict future sales, even if the data set is limited or does not contain many data points.
Cons:
- Limited for long-term forecasting: Exponential smoothing may not perform as well when applied to longer-term predictions, as it focuses on recent data and may overlook evolving trends or changes in the market.
- Assumes consistent sales patterns: This method assumes that sales patterns remain relatively stable over time. If the underlying patterns change significantly or the market dynamics shift, the forecasts generated through exponential smoothing may not accurately reflect the new reality.
- Sensitivity to initial values: Exponential smoothing forecasts can be sensitive to the selection of initial values or parameters. Small changes in the initial values can have a significant impact on the resulting forecasts, requiring careful consideration and analysis during the forecasting process.
- Lack of consideration for external factors: Exponential smoothing focuses primarily on internal historical sales data and may not explicitly account for external factors, such as changes in the competitive landscape, market conditions, or customer preferences. Incorporating external factors into the forecasting process may require additional analysis or alternative forecasting methods.
Criteria for selecting the right method
How do you decide which forecasting method is best for your business? To choose a model that aligns with your needs and circumstances, you should consider several criteria, such as:
- Data availability: Evaluate the availability, quality, and quantity of past data you have. Some sales forecasting models, such as time series and regression analyses, rely heavily on historical data. If you have a robust dataset, these quantitative methods may be suitable. On the other hand, if historical data is limited or unreliable, qualitative methods that rely on expert opinions or market research may be more appropriate.
- Forecasting horizon: Determine the time frame for which you need to forecast. Short-term forecasts (days, weeks, or months) may require different methods than long-term forecasts (quarters, years, or beyond). Time series analysis and exponential smoothing are commonly used for short-term forecasts, while regression analysis and market research can be more effective for long-term predictions.
- Forecasting accuracy: Assess the desired level of accuracy for your sales forecasts. Quantitative methods, such as time series or regression analysis, tend to provide more precise numerical forecasts. Qualitative methods, such as expert opinions or market research, may offer broader insights but with a potential for higher uncertainty.
- Data patterns and relationships: Analyze your sales dataβs underlying patterns, trends, and relationships. Time series analysis or regression analysis may be suitable if you observe seasonality, trends, or clear correlations with other variables. Conversely, qualitative methods or more straightforward techniques like moving averages may be more appropriate if there are no discernible patterns or relationships.
Metrics that could help predict sales
Sales forecasting becomes much easier when you know which metrics to focus on.Β
Outbound lead generation metrics
Lead generation metrics, such as:
- the number of leads generated,Β
- conversion rates,
- lead quality,Β
directly impact sales forecasting. Analyzing these metrics helps estimate the volume of potential opportunities and the effectiveness of lead generation strategies.
But how to measure lead generation? You can do it with the help of Expandi, which is the best LinkedIn automation tool.Β
Expandi reporting tools help you understand the number of leads generated via outbound. You can use the number of sales leads, cycles, and close rates as a metric to predict future sales.
Sales funnel metrics
Sales funnel metrics track the progression of sales opportunities through different stages of the sales process. Key metrics include:
- The number of opportunities at each stage.
- Conversion rates between stages.
- Average deal size.
- Sales cycle length.
These metrics help assess the health of the sales pipeline and forecast future sales performance.
Customer acquisition cost (CAC)
CAC measures the cost associated with acquiring a new customer. It helps evaluate the efficiency of sales and marketing efforts and provides insights into the investment required to drive future sales growth. Understanding CAC is essential for accurate sales forecasting and budgeting.
Customer churn and retention rates
Customer churn and retention rates measure the percentage of customers lost over a specific period and the percentage of customers retained, respectively. These metrics directly impact revenue and should be considered in sales forecasting to account for customer attrition or retention trends.
Average sales cycle length
The average length of the sales cycle, from initial contact to closing the deal, affects sales forecasting. Longer sales cycles may delay revenue recognition, while shorter cycles can accelerate revenue generation.
Seasonality and time factors
Many businesses experience seasonal fluctuations in sales. Understanding seasonality patterns, such as peak sales periods or periods of low demand, is crucial for accurate forecasting. Additionally, considering time factors, such as month-over-month or year-over-year growth rates, helps account for temporal variations in sales performance.
Economic indicators
External economic indicators, such as GDP growth, unemployment, interest, and consumer confidence, can impact sales forecasting. These indicators provide insights into the overall economic conditions and customer spending behavior.
Best practices for sales forecasting
Sales forecasting may seem like an impenetrable jungle, but it becomes much easier if you follow some proven practices. Letβs take a look at some of them.
Involve sales teams in forecasting
Involving sales teams in forecasting is crucial to predict customer demand and sales opportunities accurately. They are on the front line of customer interactions. They have direct knowledge of customer preferences, buying behavior, and market trends.
Moreover, sales teams can provide valuable real-time feedback on market conditions, competitive dynamics, and customer sentiment. With this feedback, you can adjust forecasts based on changing market dynamics and ensure that projections align with current realities.
Engaging sales teams in forecasting allows for a more comprehensive evaluation of sales performance. By comparing actual results against forecasted targets, sales teams can assess their performance, identify improvement areas, and adjust strategies accordingly.
Use strategies to improve sales forecast accuracyΒ
To improve the accuracy of sales forecasting, consider implementing the following strategies:
- Historical Data Analysis: Analyze historical sales data to identify patterns, trends, and seasonality. Look for consistent sales cycles, growth rates, and other factors that can serve as a basis for future projections. Historical data provides valuable insights into customer behavior and market dynamics, allowing for more accurate forecasts.
For example, Expandi gives you access to statistics where you can analyze the response rate of your messages in every particular period. Knowing this, you can adjust settings to send more or fewer connection requests and follow-up messages, which, in turn, will allow you to predict future sales.
- Segment and Validate Data: Break down sales data into meaningful segments based on product lines, customer segments, geographic regions, or other relevant factors. Analyze each segment separately to understand variations in performance and apply appropriate forecasting methods.
- Use Multiple Forecasting Methods: Employ a combination of qualitative and quantitative forecasting methods. Qualitative methods like market research and expert opinion, provide insights into market trends and customer preferences. Quantitative methods, like time series or regression analysis, leverage historical data and statistical techniques to make predictions. By using multiple strategies, you can cross-validate forecasts and obtain a more comprehensive view.
- Incorporate External Factors: Consider external factors that can impact sales, such as changes in the market, economic indicators, industry trends, or competitive landscape. Monitor and analyze these factors to incorporate them into the forecasting process and adjust projections accordingly.
Regularly review and update forecasts
Regularly reviewing and updating forecasts is crucial for maintaining the accuracy and relevance of sales projections. Markets are dynamic, and factors influencing sales performance can change rapidly. Businesses must review and update forecasts regularly to incorporate the latest data and market insights into their projections. It ensures that forecasts reflect the most current realities and provides a more accurate basis for decision-making.
External factors such as economic conditions, industry trends, and competitive landscape can also significantly impact sales. Organizations must regularly review and update forecasts to respond to these changes effectively.
They can adjust sales strategies, allocate resources appropriately, seize emerging opportunities or mitigate potential risks.
Tools for Sales Forecasting
To further improve sales forecast accuracy, consider using the following sales forecasting tools:
Customer relationship management (CRM) software
CRM platforms like Salesforce, HubSpot CRM, or Zoho CRM often include built-in forecasting functionalities. These tools allow sales teams to track and manage customer interactions, sales opportunities, and revenue projections. CRM systems provide a centralized database for storing and analyzing sales data, enabling more accurate forecasting.
Sales analytics software
Sales analytics tools like Tableau, Microsoft Power BI, or InsightSquared provide advanced analytics and reporting capabilities. They integrate with CRM systems and other data sources to analyze sales performance, identify trends, visualize data, and generate forecasts based on historical patterns and predictive algorithms.
Sales engegement platforms
Sales engagement platforms like Hubspot Sales Hub, Groove or Expandi can also help predict future sales.
Expandi is intelligent software that focuses on LinkedIn automation and sales outreach. It alleviates the burden of creative thinking and assists in generating content tailored to your audience.
Expandi can help you forecast sales by using such LinkedIn sales analytics like profile views, post views and content reach, search appearances, social index, outreach campaigns and lead generation stats.
Excel Spreadsheets
Excel is a widely used tool for sales forecasting due to its flexibility and familiarity. It allows businesses to create custom forecasting models using formulas, charts, and data analysis techniques. Excel templates and add-ons designed explicitly for sales forecasting provide pre-built frameworks to simplify the process.
Forecasting Software
Dedicated forecasting software solutions are also available. They include such tools as Anaplan, Adaptive Insights, or Demand Works Smoothie. These tools offer sophisticated forecasting capabilities, enabling businesses to create comprehensive sales forecasts by incorporating historical data, market trends, and other variables. They often include scenario modeling, collaboration, and advanced forecasting algorithms.
Conclusion
Accurate sales forecasting is a critical ingredient in the recipe for business success. As organizations navigate the ever-changing landscape of customer demands, market dynamics, and internal operations, choosing appropriate metrics becomes paramount in predicting sales outcomes. In this article, we have explored various sales forecast methods and the metrics that can unlock valuable insights.
In the dynamic and competitive business landscape, the ability to predict sales outcomes with precision is a strategic advantage. By harnessing the right metrics and continuously refining forecasting practices, businesses can position themselves for success, unlock new opportunities, and achieve their sales targets in the ever-evolving marketplace.
Even with the most accurate sales forecasts, things may go differently than your plan. But there’s a solution in such a situation: you can use the potential of LinkedIn to generate B2B leads. Expandi allows you to utilize intelligent and automated sequences to reach potential customers through email or LinkedIn. It enables you to swiftly initiate your marketing campaign within just 15 minutes and obtain valuable outcomes within a 24-hour timeframe. Want to check how it works? Sign up for Expandi free trial to unlock the power of LinkedIn prospecting.
FAQ
What are the 3 forecasting techniques?
Among several sales forecasting techniques, three are the most common. They include:
- Time Series Analysis is a forecasting technique that involves analyzing historical data to identify patterns, trends, and seasonality in the data. Time series analysis uses statistical methods to forecast future values based on the historical patterns observed. It assumes that future behavior will follow similar patterns as seen in the past.
- Regression analysis involves identifying the relationship between the variable to be forecasted (dependent variable) and one or more other variables (independent variables). This sales forecasting technique uses historical data to develop a regression model and estimate the future values of the dependent variable based on the values of the independent variables. Regression analysis can be simple (with one independent variable) or multiple (with multiple independent variables).
- Qualitative forecasting techniques rely on expert judgment, opinions, or market research to predict future outcomes. These techniques are often used when historical data is limited or when there are significant changes in market conditions or customer preferences. Qualitative forecasting methods include the Delphi method, market research, surveys, and expert opinions.
It’s important to note that these techniques can be used individually or in combination, depending on the specific forecasting requirements and data availability.
What are the 2 main methods of forecasting?
The two main methods of forecasting are:
Qualitative Forecasting: Qualitative forecasting methods rely on subjective judgments, opinions, and expert insights to predict future outcomes. These methods are typically used when historical data is limited, unreliable or when significant changes in market conditions or customer preferences are expected. Qualitative forecasting methods include market research, surveys, expert opinions, the Delphi method, and scenario analysis. These techniques involve gathering information from various sources, analyzing qualitative data, and making predictions based on subjective assessments.
Quantitative Forecasting: Quantitative forecasting methods rely on historical data and statistical models to predict future outcomes. These methods are based on the assumption that historical patterns and relationships can be used to forecast future values. Quantitative forecasting techniques include time series analysis, regression analysis, exponential smoothing, moving averages, and Box-Jenkins models. These methods involve analyzing historical data, identifying patterns, trends, seasonality, and other factors to generate numerical forecasts.
Both methods have their strengths and weaknesses. The choice of method depends on several factors like:
- The availability and quality of data
- The nature of the forecasted variable
- The time frame of the forecast
- The specific requirements of the forecasting task
Youβve made it all the way down here, take the final step